What is an Identity Graph? Unify Customer Data for Smarter Insights
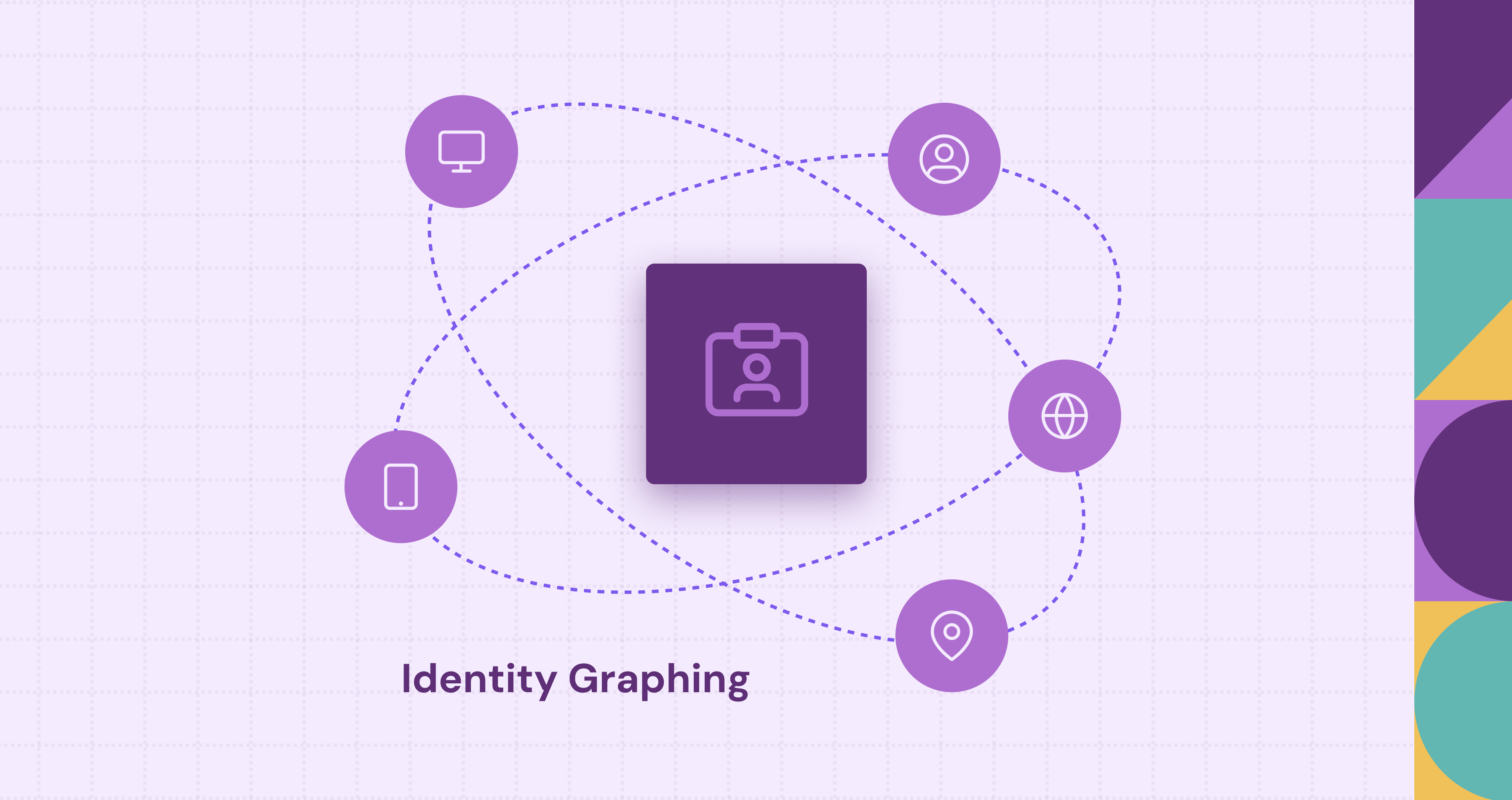
There are lots of ways that people interact with businesses. Whether it be through websites, mobile apps, social media, physical stores, or customer service channels, these potential and existing customers interact using multiple devices and identifiers. This fragmentation leads to a lot of siloed data, which prevents personalization, accurate measurement of marketing effectiveness, or detection of sophisticated fraud. An identity graph is the answer that connects these disparate data points to create a single and accurate view of each person. This post explains what identity graphs are, how they work, their components, use cases, technologies involved, challenges, and role in data strategy. Let's begin by exploring the core of what an identity graph is.
What is an identity graph?
An identity graph is a database that maps and links various identifiers associated with individuals back to a single profile. Based on graph theory fundamentals, it represents identifiers as nodes and their relationships as edges. Think of it as a system that collects signals, represented as email addresses, phone numbers, cookie IDs, device IDs, IP addresses, account logins, customer IDs, from different touchpoints and determines which ones belong to the same person or entity (like a household). The goal is to resolve identities across multiple platforms and devices, creating a stable, complete view of each user or customer.
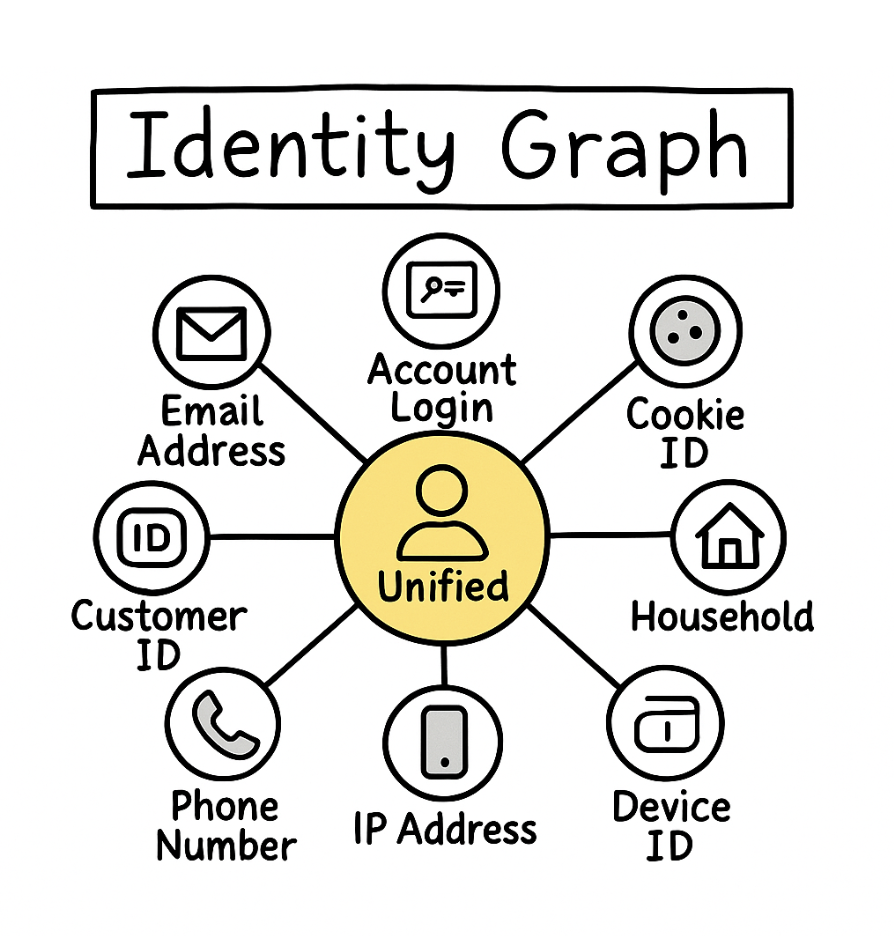
This is in contrast to traditional data storage, where customer information might live in separate databases (CRM, web analytics, mobile app backend, marketing automation) with no way to link them together. By stitching this data together, an identity graph becomes a central hub and source of truth.
When it comes to identity graphs, there are two main approaches to identity resolution:
- Deterministic Matching: This method relies on known, confirmed links between identifiers. Examples include a user logging into a website with an email address (linking the email to a cookie or device) or using a verified phone number across multiple services. Deterministic matches offer high accuracy (nearly 100% confidence) because they are based on explicit associations, often provided directly by the user.
- Probabilistic Matching: When deterministic links are not available, this approach utilizes algorithms and statistical models to infer connections. It analyzes patterns in non-explicit data points, such as IP addresses, device types, operating systems, browser user agents, location data, and browsing patterns. If multiple signals consistently co-occur, the system assigns a probability score indicating the likelihood of a match. This method expands reach but involves uncertainty, and requires careful threshold setting (often 80% confidence or higher) to balance reach and accuracy.
Many modern identity graphs use a hybrid approach, prioritizing deterministic matching for accuracy and supplementing with probabilistic matching to increase the number of connected profiles and identifiers.
How identity graphs work
Similar to other graph implementations, such as more traditional knowledge graphs, building and maintaining an identity graph involves several key processes. These processes include:
Data Ingestion
The process begins by collecting identifier data from various sources: first-party (collected directly), second-party (shared from partners), and potentially third-party data (from data aggregators, although use is declining due to privacy concerns). This involves handling high volumes of data, often requiring distributed stream processing systems to manage the throughput from websites, apps, CRM, offline sources, etc.
Identity Resolution
Next, all of this data needs to run through the core engine, applying identity resolution algorithms to match ingested identifiers based on anonymous and known data. As mentioned before, the two main algorithms are applied by identity resolution solutions include:
- Deterministic algorithms search for exact matches using known identifiers, typically comparing securely hashed values (e.g., hashed email addresses or phone numbers) to safeguard sensitive information.
- Probabilistic algorithms analyze patterns among non-definitive identifiers. Machine learning models, often ensemble methods that combine techniques such as logistic regression, random forests, or neural networks, are frequently used to calculate confidence scores for inferred links based on correlations in attributes like IP addresses, device characteristics, and behavior.
Graph Construction
As identifiers are matched, the identity graph is built, commonly using graph database technology optimized for relationship analysis. This data is then pushed into the corresponding nodes and edges within the graph. Nodes (Vertices) represent individual identifiers (email addresses, device IDs) or unified identity profiles, and edges (Links) represent connections between identifiers, attributed with details such as match type, confidence score, and timestamp.
With the data in place, graph query languages (like Cypher or Gremlin) can then be used to traverse these connections. For example, graph users can then use simple Cypher queries like the one below to find all devices associated with a specific email:
// Find all devices linked to a specific email hash
MATCH (e:EmailIdentifier {value: "5f4dcc3b5aa765d61d8327deb882cf99"})
<-[:HAS_IDENTIFIER]-(p:Profile)-[:HAS_IDENTIFIER]->(d:DeviceIdentifier)
RETURN d.type, d.value, d.last_seen
Storing the data in a graph enables complex queries like finding all devices linked to a known customer or segmenting users based on connected attributes. These types of queries border on impossible when data is siloed across multiple traditional data technologies, especially SQL-based ones.
Graph Maintenance
Far from “build once and forget about it”, Identity graphs are dynamic and require ongoing updates. Key aspects of identity graph maintenance include:
- Real-time or near real-time updates to incorporate new interactions and identifiers rapidly.
- Link validation and decay processes to periodically re-evaluate probabilistic links and remove outdated or low-confidence connections, sometimes using time-to-live (TTL) settings.
- Efficient mechanisms for handling data privacy requests (access, deletion) across the distributed graph.
- Persistence strategies to maintain unified profiles even as individual identifiers change or expire.
- Carefully designed indexing on key identifiers and properties is crucial for query performance, especially for real-time applications.
Similar to other forms of real-time graph analysis, these tasks allow the identity graph to grow while staying up-to-date and accurate.
Key components of an identity graph
Within the identity graph itself, there are quite a few moving parts. A functional identity graph consists of components of the underlying graph structure (nodes and edges) but also many other facets that help make the graph secure, accurate, and accessible. At the graph database level, we have:
- Identifiers (Nodes)
- These are the raw data points collected. Examples include:
- Personally Identifiable Information (PII): Email addresses, phone numbers, names (hashed or tokenized for privacy).
- Device Identifiers: Mobile Ad IDs (IDFA, GAID), vendor IDs, device fingerprints.
- Web Identifiers: First-party cookies, IP addresses, browser user-agent strings. (Third-party cookies are declining).
- Account Identifiers: User IDs, CRM IDs, loyalty numbers.
- Offline Identifiers: Hashed PII collected offline, store card numbers.
- Pseudonymous Identifiers: System-generated IDs representing unified profiles without exposing raw PII.
- These are the raw data points collected. Examples include:
- Linkages (Edges)
- These are the connections between identifiers. Attributes often include match type, confidence score, timestamp, and data source.
- Attributes/Data Points
- These are additional information associated with the unified identity profile, including: demographics, behavioral data (site visits, purchase history), technographics (devices, OS), consent status/preferences.
Beyond this underlying data, we have components that enhance accuracy, security, and accessibility. These include:
- The Resolution Engine component applies an identity resolution framework and algorithms for deterministic and probabilistic matching, processes data, calculates probabilities, and updates the graph.
- The Data Governance Layer handles critical tasks for privacy compliance and data security. Within this layer, we have:
- Consent Management: Tracking user permissions across identifiers and purposes.
- Data Access Control: Defining and enforcing who can access graph data.
- Data Protection: Masking, hashing, or tokenization for sensitive data.
- Handling Privacy Requests: DSR processes under GDPR, CCPA, etc.
- Audit Trails: Logging data access and changes.
- Lastly, we have the Query Interface/API that allows applications and analysts to interact with the graph via RESTful APIs, GraphQL endpoints, native graph query languages, or batch processing interfaces for analytics.
Although this covers all the major components, each implementation will be slightly different and may include additional components as well. Overall, designing an identity graph that incorporates all these components should ensure you end up with accurate and secure data that can be easily accessed and utilized within your organization.
Use cases of identity graphs
When are identity graphs useful? Identity graphs support various use cases across multiple industries. Let’s take a look at some of the areas where they are used to empower organizations:
Marketing and Advertising
In the marketing and advertising space, identity graphs are a key component that almost every industry leverages. They help to power:
- Personalization: Deliver experiences tailored to the comprehensive picture of user preferences and behavior.
- Cross-Device Targeting: Reach the same user across their devices.
- Frequency Capping: Control ad exposure accurately across devices.
- Measurement and Attribution: Track the customer journey across touchpoints, improve marketing effectiveness. These often require graph traversals to identify related identifiers and attributes for segmentation or targeting in near real-time.
- Audience Segmentation: Create more precise segments based on unified profiles. For example, organizations can use Cypher queries like the one below to find high-value customers who have recently browsed a specific product category:
// Find high-value customers interested in premium products
MATCH (u:UserProfile)-[:HAS_ATTRIBUTE]->(a:Attribute)
WHERE a.lifetime_value > 1000
MATCH (u)-[:HAS_IDENTIFIER]->(c:CookieID)-[:HAS_EVENT]->(e:PageView)
WHERE e.product_category = "premium"
AND e.timestamp > timestamp() - 7776000 // Last 90 days
RETURN u.id, count(e) as engagement_score
ORDER BY engagement_score DESC
LIMIT 1000
Customer Experience (CX)
Similar to marketing and advertising use cases, customer experience can also be augmented with the capabilities of an identity graph. Within this segment, uses include:
- Omnichannel Consistency: Recognize customers across online, mobile, and offline channels. Support agents can access relevant interaction history from the unified profile.
- Customer Journey Mapping: Understand how users navigate different channels over time.
- Proactive Support: Identify potential issues based on behavior patterns.
Cybersecurity and Fraud Detection
Another common area that leverages identity graphs is cybersecurity and fraud detection use cases. Here, you’ll see it used for:
- Identity Verification: Correlate signals across identifiers to strengthen authentication.
- Fraud Detection: Link seemingly unrelated fraudulent actions or accounts to common entities or patterns.
- Account Takeover Prevention: Detect anomalous login attempts or device usage associated with known profiles.
- Risk Scoring: Use graph connections and attributes to score user or transaction risk. Security applications often require very low latency for real-time decision-making.
Data Management and Analytics
Slightly more generic, the data management and analytics space is also heavily dependent on identity graphs to supply capabilities such as:
- Single Customer View (SCV): Establish a trusted source of truth for customer data.
- Data Enrichment: Connect disparate datasets via the unified identity.
- Better Analytics: Analyze behavior, lifetime value, and segmentation more accurately using unified data. Advanced graph algorithms uncover hidden patterns.
Compliance and Privacy
Lastly, and core to many businesses, is the use of identity graphs in compliance and security. With the growing number of compliance and privacy requirements (from legislation such as GDPR), identity graphs are used for:
- Consent Management: Link consent gathered on one channel to the entire user profile.
- Data Subject Requests (DSRs): Find all data associated with an individual across systems for access or deletion requests.
Identity graph use cases stretch far and wide. This breakdown illustrates the impact of identity graphs on various capabilities utilized by modern businesses. Next, it’s time to explore the technologies and platforms that enable businesses to develop these capabilities.
Identity graph technologies & platforms
There are several ways to set up identity graphs within an organization. Many build their identity graphs in-house or use vendor services to scale them out quickly. The “how” of creating an identity graph depends on expertise, budget, scale, control requirements, and time-to-market requirements.
Building an identity graph involves:
- Graph Databases or Query Engines: Technologies such as PuppyGraph, Neo4j, TigerGraph, ArangoDB, and AWS Neptune are utilized due to their efficiency in managing and querying highly connected data. The choice depends on scale, performance needs, and query language.
- Distributed Data Processing Frameworks: Apache Spark or Flink are often used to handle large-scale data ingestion, transformation, and complex matching algorithms in batch or real-time.
- Machine Learning Libraries: Used to develop and train probabilistic matching models (e.g., TensorFlow, PyTorch, scikit-learn).
- Data Warehousing/Lakehouse Platforms: Platforms like Snowflake, BigQuery, or Databricks serve as the underlying storage and processing layer, integrating with graph engines or identity tools. Managing graphs with billions of nodes and edges often requires advanced techniques, such as data partitioning (sharding), to distribute the data and workload across a cluster.
Several platforms offer identity graph capabilities:
PuppyGraph
PuppyGraph is the first and only real time, zero-ETL graph query engine in the market, empowering data teams to query existing relational data stores as a unified graph model in under 10 minutes, bypassing traditional graph databases' cost, latency, and maintenance hurdles. Being the first graph query engine, it is the perfect tool for building identity graphs quickly and at scale. Using your underlying data infrastructure, PuppyGraph can connect to SQL and NoSQL data sources and map the data into a graph. The data used to power the graph is derived from the underlying data sources, and no ETL or sophisticated pipelines are required. In a matter of minutes, you can map your data into an identity graph that enables lightning-fast query performance and is highly scalable.
PuppyGraph seamlessly integrates with major relational databases like PostgreSQL and MySQL. It also supports data lakes like Apache Iceberg and Delta Lake. PuppyGraph’s native graph analytic engine gives you sub-second query execution. Its high-performance capabilities remain consistent, which helps users achieve petabyte-level scalability in their data systems without costly infrastructure changes.
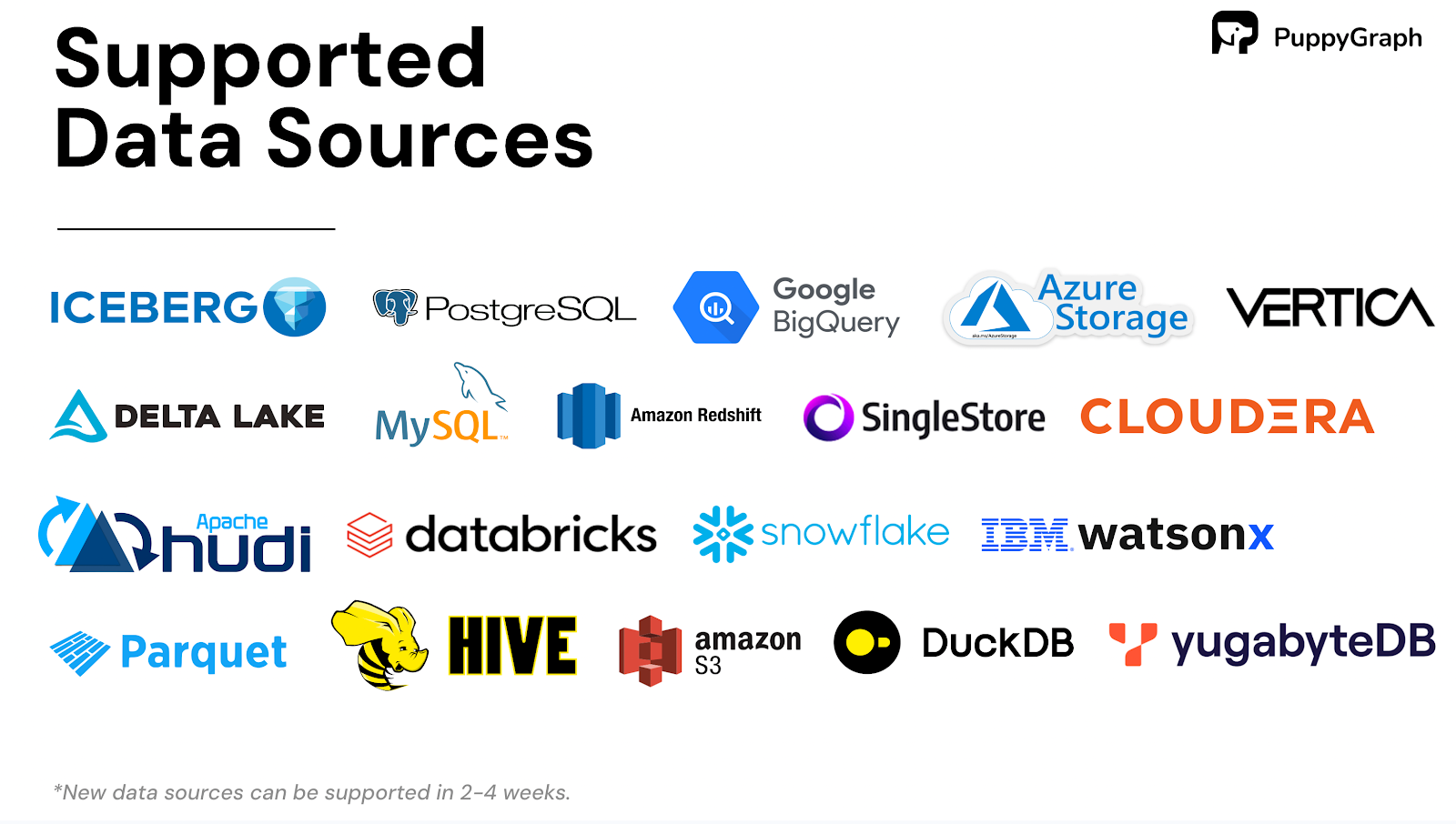
PuppyGraph’s flexible data model accommodates various data relationships. It gives you a range of both automated and manual graph modeling tools that can efficiently translate SQL data into a graph representation. Additionally, PuppyGraph automatically proposes optimal mapping strategies for data points. You get the best user experience with guided support and automation in model development.
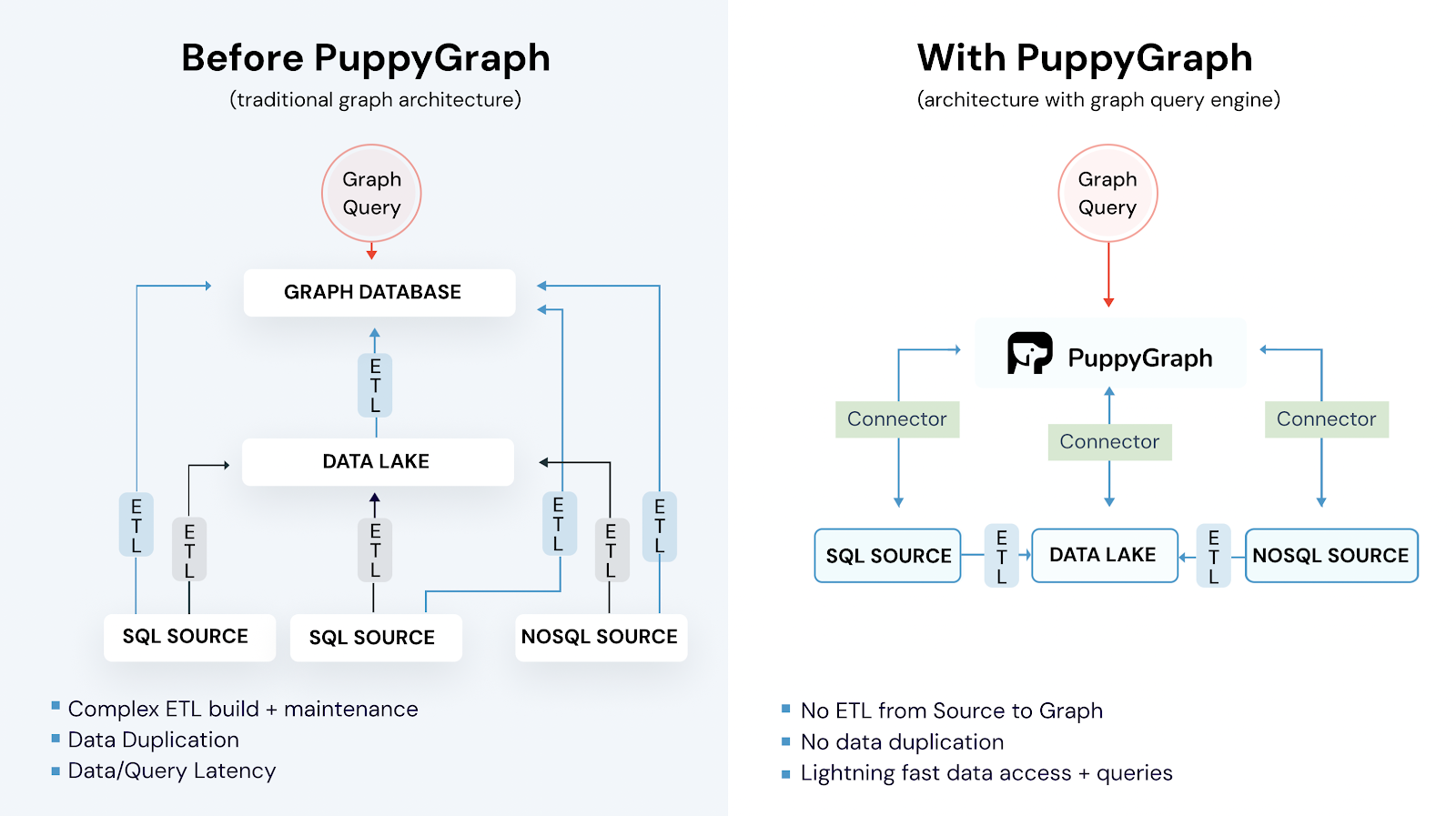
PuppyGraph also gives you a centralized hub for graph data visualization. With its wide array of data sources support, it doesn’t matter where and how your data resides—plain text, databases, or data warehouses. You don’t have to go through the hassle of hopping between tools to visualize and analyze data for different data sources. All data converges into a single graph that becomes the single source of truth for your visualization and analytics processes through PuppyGraph’s user-friendly platform.
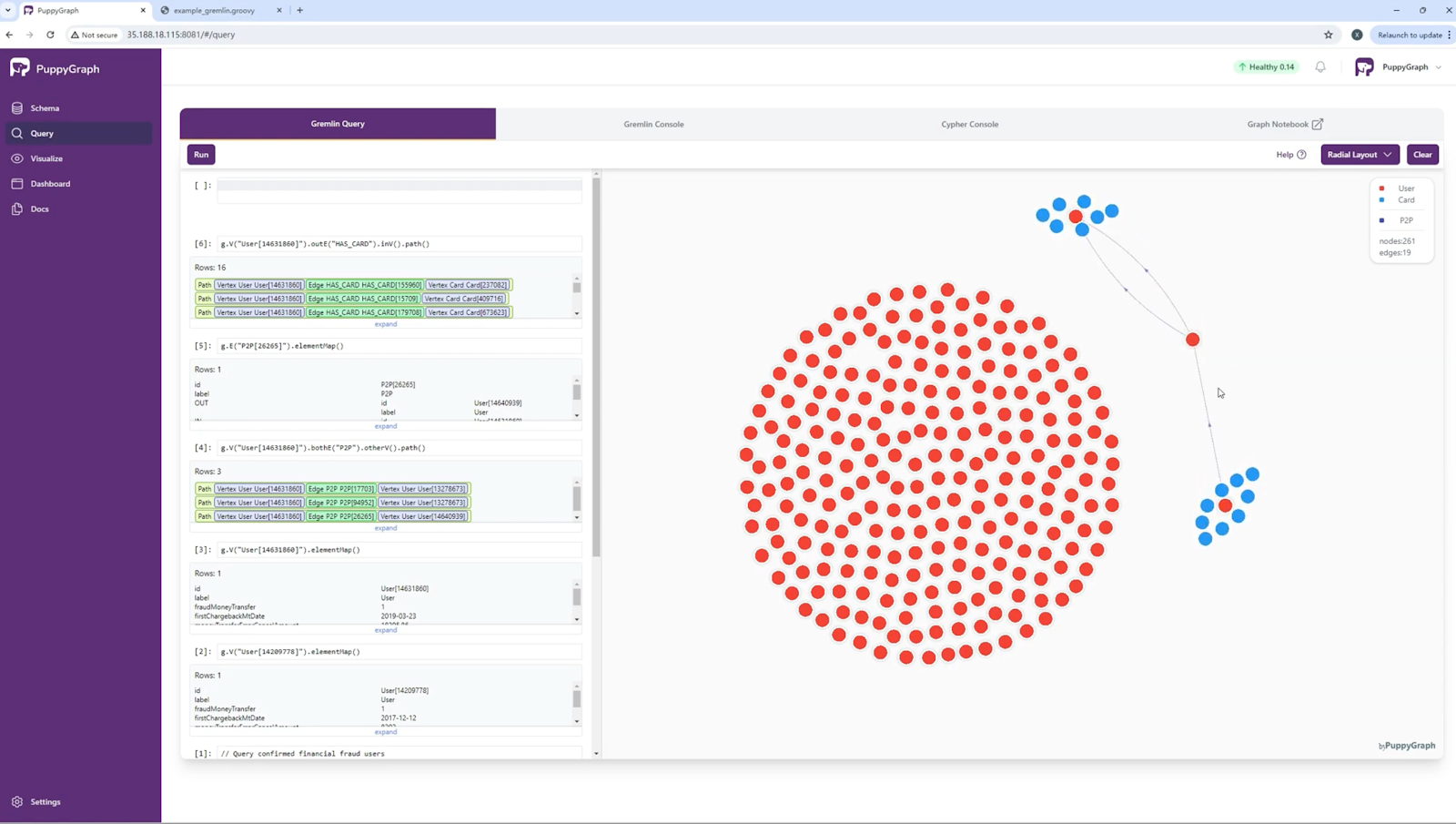
LiveRamp IdentityLink
A data connectivity platform providing a widely adopted identity graph as a service. It focuses on connecting data across ecosystems, mainly for marketing, with privacy and data clean rooms built on its identity infrastructure.
Adobe Experience Platform Identity Service
Part of Adobe Experience Cloud, this service provides a framework for stitching customer identities within private graphs specific to an organization. It focuses on real-time profiles based on first-party data to power personalization within the Adobe ecosystem and beyond.
Snowflake (via Ecosystem or Native Capabilities)
As a cloud data platform, Snowflake enables the building of identity graphs using partner tools (via the Snowflake Marketplace or by using tools such as PuppyGraph to expose graph capabilities) or custom logic. Its architecture supports large-scale storage, processing, and secure data sharing (including clean rooms), so it's a viable foundation for identity resolution.
Although not an exhaustive list, these solutions are the most popular ones that businesses choose to start with much of the time. They are proven solutions that allow organizations to create identity graphs that scale. Regardless of the technology, there are still some things to be aware of in terms of challenges and limitations revolving around identity graphs. Let’s look at those next.
Challenges & limitations
Building identity graphs presents numerous challenges. Although not impossible to overcome, some represent major hurdles that impact the adoption of the technology. Whether it be at the compliance, accuracy, or scalability levels, there are multiple facets to consider when choosing to create an identity graph and when selecting the underlying technologies to build it on. Here are some areas to focus on:
Data privacy and regulations
Navigating global regulations, such as the GDPR and CCPA, is a key factor for many businesses considering the implementation of an identity graph. This involves establishing legal bases for processing (such as consent), accurately managing preferences, honoring data subject rights, implementing robust security measures, and adapting to ecosystem changes, including the deprecation of third-party cookies and mobile ID restrictions, thereby increasing reliance on first-party data.
Accuracy and scale
Maintaining high matching accuracy, especially with probabilistic methods, is hard. False positives/negatives degrade value. Scaling the system to handle potentially billions of identifiers and connections while maintaining performance and accuracy requires advanced engineering, significant computational resources, and well-engineered optimization techniques, including partitioning and sharding.
Identity ambiguity
Distinguishing individuals on shared devices or within households based solely on digital signals is challenging, such as when they are using the same IP address. Users may have separate online personas. These ambiguities challenge resolution models and can lead to inaccuracies or data remaining anonymous, as it cannot be accurately matched.
Identifier decay and volatility
Many identifiers, such as cookies, mobile IDs, and IP addresses, are ephemeral. The graph must constantly ingest updates and manage identifier lifespan to remain accurate over time. Failure to do this in a timely and consistent manner means that the usefulness of the data within the graph is potentially flawed.
Security considerations
Concentrating sensitive linked data makes identity graphs a target. Minimizing the graph's attack surface and applying security practices are essential, including end-to-end encryption, field-level protection for sensitive data, strict access controls (principle of least privilege), regular security audits, and comprehensive monitoring. Although this is easy to do in principle, with the vast array of security technologies available, the threat landscape is constantly changing, and solutions must keep up to truly keep attackers out. As many are aware, staying ahead of the curve in terms of security is not always possible, presenting a constant challenge.
Cost and complexity
Lastly, and likely of most importance to businesses considering using an identity graph, is the fact that building and maintaining an identity graph requires significant investment. This includes expanding the budget for technology, infrastructure, and specialized expertise, including data engineering, data science, graph databases, and privacy. Using vendor platforms also comes with costs and integration challenges. The cost of creating and maintaining an identity graph can vary widely, with many tradeoffs depending on the technologies and scale required to make something of actual value for the business.
Conclusion
Identity graphs solve a critical challenge: linking user identifiers across devices and platforms to create a complete, accurate view of each individual. They power personalized marketing, better customer experiences, stronger security, and smarter analytics—but building them comes with challenges around privacy, scale, and integration.
PuppyGraph makes it easy to create high-performance identity graphs without ETL. Connect directly to your data sources and start querying in minutes. Download our free Developer Edition or book a free demo to see how PuppyGraph can help you move faster.
Get started with PuppyGraph!
Developer Edition
- Forever free
- Single noded
- Designed for proving your ideas
- Available via Docker install
Enterprise Edition
- 30-day free trial with full features
- Everything in developer edition & enterprise features
- Designed for production
- Available via AWS AMI & Docker install